Predictive maintenance in food industry
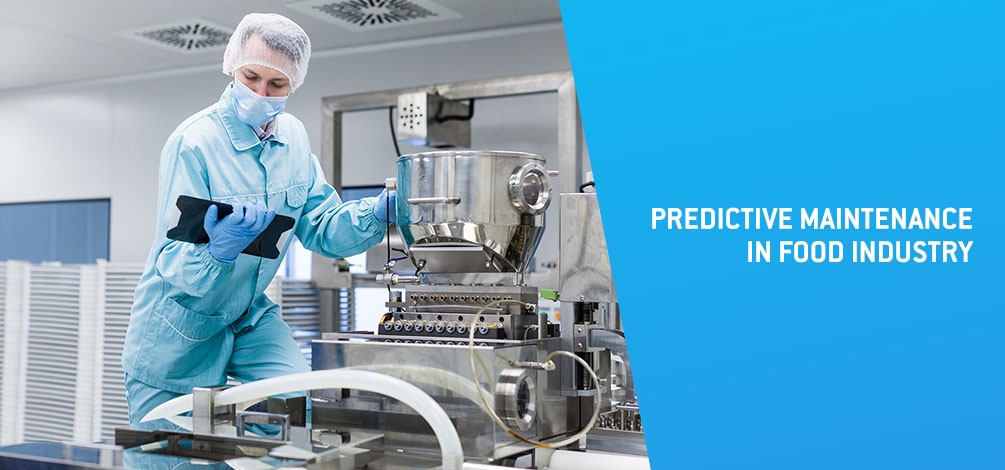
The contribution of predictive maintenance is extended to all industrial sectors, but in the food industry the contribution reveals an added value, which has a special relevance in the final product.
The maintenance methodology of an industrial unit is an essential part, in those that are the objectives of performance and productive efficiency. Continuous monitoring is increasingly as an essential part of optimizing maintenance interventions.
Among the most familiar maintenance models in the industry, predictive maintenance is evidenced by giving a new use to data, collected from machines and the surrounding environment. As the name suggests, this model is based on the prediction of the occurrence of scenarios or possible failures that may interfere with the normal functioning of the entire production process chain. The contribution of predictive maintenance is extended to all industrial sectors, but in the food industry the contribution reveals an added value, which has a special relevance in the final product.
The existing regulation of the food industry aims to ensure that the final product respects the required quality standards. The modernization of the food industry and production equipment has allowed to increase production capacity and efficiency, however, the complexity of processes may mean a significant increase in downtime inherent to equipment failures and maintenance. The condition monitoring of the equipment has an added relevance, since it is from there that the necessary data to define and schedule maintenance interventions are extracted, with the least possible impact on the level of resources, whether at the mechanical level or at time needed for technical intervention.
Figure 1 - Variables in production equipments
Over the production process, it is necessary to define and implement a monitoring model that allows the collection and visualization of data, supported by the assignment of operating limits on the several variables inherent to the process. The correlation of the variables allows detecting, in a time, deviations in the process, which can interfere with the normal operation of the machines and, consequently, have a direct impact on the quality rates of the products. The existence of an alarming model associated with the data collected in real time, values the entire process and ensures that maintenance can be planned and carried out in a preventive phase, instead of being carried out in a corrective phase often characterized by longer downtime and increased costs.
Figure 2 - Limit values and notification actions
Stopping a production line in the food industry is clearly different from stopping a production line for non-perishable products. Despite safety being a priority in these two scenarios, if the production of parts is interrupted, there will be no deterioration of the parts until the equipment returns to normal operation and the losses will only be attributed to the production time. In a stop at the food production unit, the ingredients and other compounds may change, jeopardizing the quality of the final product, noting losses both in terms of product and production time. More than affecting product quality, it will affect the consumer experience and, in more complex situations, involve legal issues with brands and distributors.
Tekon Electronics's PLUS wireless solutions have been streamlining data collection strategies in the context of the food industry, where variables such as temperature, humidity, rotation, vibration, pressure, among others, allusive to the production process and can affect quality of product. In this way, is obtained a greater visibility of the reference points in the infrastructure and process.
With the arise of the new industrial revolution, Industrial Internet of Things (IIoT), the digitization of production processes is promoted by sensors and equipment that ensure the collection and storage of data locally or, more often, in the cloud. Convergence to cloud solutions allows greater user connectivity and information security, enabling access to platforms and data, regardless of user’s location and devices.
Figure 3 - Integration of PLUS system on Tekon IoT Platform
IoT platforms are increasingly becoming a reality in this context, where the graphical visualization of the data and the monitoring component is highlighted for the user. Tekon IoT Platform from Tekon Electronics, allows the collection, processing, visualization, and advanced analysis of the data, promoting an assertive decision making, regarding the scheduling of maintenance events.
Figure 4 - Advanced data analytics
Predictive maintenance contribution
Predictive maintenance proves to be an economically viable strategy for the food industry, resulting in reduced costs of maintenance procedures and increased production time. The major contribution of predictive maintenance to the food industry can be concentrated in two parts: productive efficiency and operational performance. The first involves maintaining productive equipment with high OEE (Overall Equipment Effectiveness) indexes, where ideal operating conditions are verified. Operational performance represents the presentation of a final product with consistent quality standards, capable of continuing to earn consumer confidence.
OEE = Availability x Performance x Quality
Predictive maintenance is a clear example of how data should be structured and prepared to be assimilated by Machine Learning and Artificial Intelligence applications. At Tekon Electronics, the development of industrial solutions is considered based on this premise, in which data can always be processed and structured for rapid integration in these contexts.